
However, the small confidence bounds do not cross zero on p1, p2, and p3 for the quadratic fit, indicating that the fitted coefficients are known fairly accurately. However, as the polynomial degree increases, the coefficient bounds associated with the higher degree terms cross zero, which suggests over fitting. The fitted coefficients associated with the constant, linear, and quadratic terms are nearly identical for each normalized polynomial equation. If the higher order model terms may have coefficients of zero, they are not helping with the fit, which suggests that this model over fits the census data. This means you cannot be sure that these coefficients differ from zero. The bounds cross zero on the p1, p2, and p3 coefficients for the fifth-degree polynomial. Do not compare normalized coefficients directly with non-normalized coefficients. Note that p2 refers to the p2*x term in 'poly2' and the p2*x^4 term in 'poly5'. ) to see the model terms for each coefficient. The confidence bounds on the coefficients determine their accuracy. Resolve the best fit issue by examining the coefficients and confidence bounds for the remaining fits: the fifth-degree polynomial and the quadratic.Įxamine population2 and population5 by displaying the models, the fitted coefficients, and the confidence bounds for the fitted coefficients: Which one should you choose? Compare the Coefficients and Confidence Bounds to Determine the Best Fit However, the SSE and adjusted R-square values for the remaining polynomial fits are all very close to each other. The next best SSE value is associated with the fifth-degree polynomial fit, 'poly5', suggesting it might be the best fit. However, the behavior of this fit beyond the data range makes it a poor choice for extrapolation, so you already rejected this fit by examining the plots with new axis limits. The lowest SSE value is associated with 'poly6'. The large SSE for 'exp1' indicates it is a poor fit, which you already determined by examining the fit and residuals. The adjusted R-square statistic is generally the best indicator of the fit quality when you add additional coefficients to your model. The SSE statistic is the least-squares error of the fit, with a value closer to zero indicating a better fit. Read more about our work to promote college and career readiness.Examine the sum of squares due to error (SSE) and the adjusted R-square statistics to help determine the best fit.
#FIT MATHEMATICA FULL#
"Study of Enhanced College Advising in Upward Bound: Impacts on Steps Toward College." Full Report | Appendices | Study Snapshot | Study Highlights Read Study of Enhanced College Advising in Upward Bound: Impacts on Steps Toward College by Alina Martinez, Tamara Linkow, Hannah Miller, and Amanda Parsad. The next report from the study will examine whether and where students attend college as well as the selectivity of the colleges they attend.


“Future reports will look at students’ college enrollment choices.” “This early look at Find the Fit shows some encouraging signs of changing students’ behavior in applying to colleges,” notes Senior Researcher Alina Martinez, lead author of the report.

During the study, Find the Fit was made available to Upward Bound projects to be integrated into the college advising they already offered. Find the Fit helps students understand college application steps and financial aid and clarifies their aspirations and expectations related to their choice of college.
#FIT MATHEMATICA FREE#
Did not affect the share of students completing the Free Application for Federal Student Aid earlyįind the Fit bundles low-cost, research-based strategies to address some challenges students from low-income families face in applying to and choosing a college.Had no impact on the importance students placed on academic quality in choosing a college.
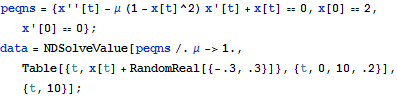
